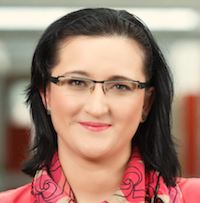
Keynote title: Representation Learning as a new Approach to Biomedical Research
Presenter: Marinka Žitnik, a postdoctoral research fellow in Computer Science at Stanford University (with Harvard University from December 2019).
Abstract
Large datasets are being generated that can transform science and medicine. New machine learning methods are necessary to unlock these data and open doors for scientific discoveries. In this talk, I will argue that machine learning models should not be trained in the context of one particular dataset. Instead, we should be developing methods that combine data in their broadest sense into knowledge networks, enhance these networks to reduce biases and uncertainty, and then learn and reason over the networks.
My talk will focus on two key aspects of this goal: representation learning and network science for knowledge networks. I will show how realizing this goal can set sights on new frontiers beyond classic applications of neural networks on biomedical image and sequence data. I will start by presenting a framework that learns deep models by embedding knowledge networks into compact embedding spaces whose geometry is optimized to reflect network topology, the essence of networks.
I will then describe two applications of the framework to drug discovery and medicine. First, the framework allowed us to, for the first time, predict the safety of drug combinations at scale. We embedded a knowledge network of molecular, drug, and patient data at the scale of billions of interactions for all medications in the U.S. Using the embeddings, the approach can predict unwanted side effects for any combination of drugs that patients take, and we can validate predictions in the clinic using real patient data. Second, I will discuss how the framework enabled us to predict what diseases a new drug could treat. I will show how the new approach can make correct predictions for many recently repurposed drugs and can operate even on the hardest, yet critical, diseases for which no good treatments exist.
I will conclude with future directions for learning over interaction data and translation of machine learning methods into solutions for biomedical problems.
Short-bio of presenter
Marinka Zitnik is a postdoctoral scholar in Computer Science at Stanford University. She will join Harvard University in December 2019. Her research investigates machine learning for sciences. Her methods have had a tangible impact in biology, genomics, and drug discovery, and are used by major biomedical institutions, including Baylor College of Medicine, Karolinska Institute, Stanford Medical School, and Massachusetts General Hospital.
She received her Ph.D. in Computer Science from University of Ljubljana while also researching at Imperial College London, University of Toronto, Baylor College of Medicine, and Stanford University. Her work received several best paper, poster, and research awards from the International Society for Computational Biology. She was named a Rising Star in EECS by MIT and also a Next Generation in Biomedicine by The Broad Institute of Harvard and MIT, being the only young scientist who received such recognition in both EECS and Biomedicine. She is also a member of the Chan Zuckerberg Biohub at Stanford.